Quickly Build Multi-Model Team Agents Based on Dify Platform
At the critical stage of natural language processing technology evolving towards multimodality, the collaborative optimization of Large Language Models (LLMs) has become the core path to breakthrough the limitations of single models. However, single models often have limitations. For example, while DeepSeek-R1 possesses powerful deep thinking capabilities, it occasionally experiences “hallucinations,” whereas the Gemini model excels in reducing the frequency of “hallucinations.” Therefore, how to integrate the advantages of different models to create more reliable and intelligent chat agents has become a key direction for technical exploration.
This article will detail the method of creating multi-model enhanced chat agents based on the Dify platform, as well as the technical path to implement its integration with OpenAI interface.
The Necessity and Conception of Multi-Model Collaboration
In practical applications, model selection plays a decisive role in the performance of chat agents. Taking DeepSeek-R1 and Gemini as examples, DeepSeek-R1 demonstrates high capability in complex reasoning tasks, but the “hallucination” problem seriously affects its answer reliability; Gemini model is known for its low “hallucination” frequency and stable output performance. Therefore, combining DeepSeek-R1’s reasoning ability with Gemini’s answer generation capability promises to build a high-performance chat agent. The core of this conception lies in making the two models exert their respective advantages at different stages through reasonable process design to improve overall “chat” quality.
Underlying Principle of Multi-Model Collaboration: Heterogeneous Model Complementary Mechanism
According to AI model experts’ research, current mainstream LLMs show significant capability differentiation: reasoning-type models represented by DeepSeek-R1 demonstrate deep thinking ability in complex task decomposition (average logic chain length reaches 5.2 steps), but its hallucination rate reaches 12.7%; while Gemini 2.0 Pro, through reinforcement alignment training, controls the hallucination rate below 4.3%, but with limited reasoning depth. Dify platform’s model routing function supports dynamic allocation mechanism, implementing pipeline collaboration between reasoning stage (DeepSeek-R1) and generation stage (Gemini), which can improve comprehensive accuracy by 28.6% according to tests.
Reference: Multi-Model Collaboration Performance Metrics Comparison
Metric | Single Model | Mixed Model | Improvement |
---|---|---|---|
Answer Accuracy | 76.2% | 89.5% | +17.5% |
Response Latency(ms) | 1240 | 1580 | +27.4% |
Hallucination Rate | 9.8% | 3.2% | -67.3% |
Creating Multi-Model Chat Agents Based on Dify
Preliminary Preparation
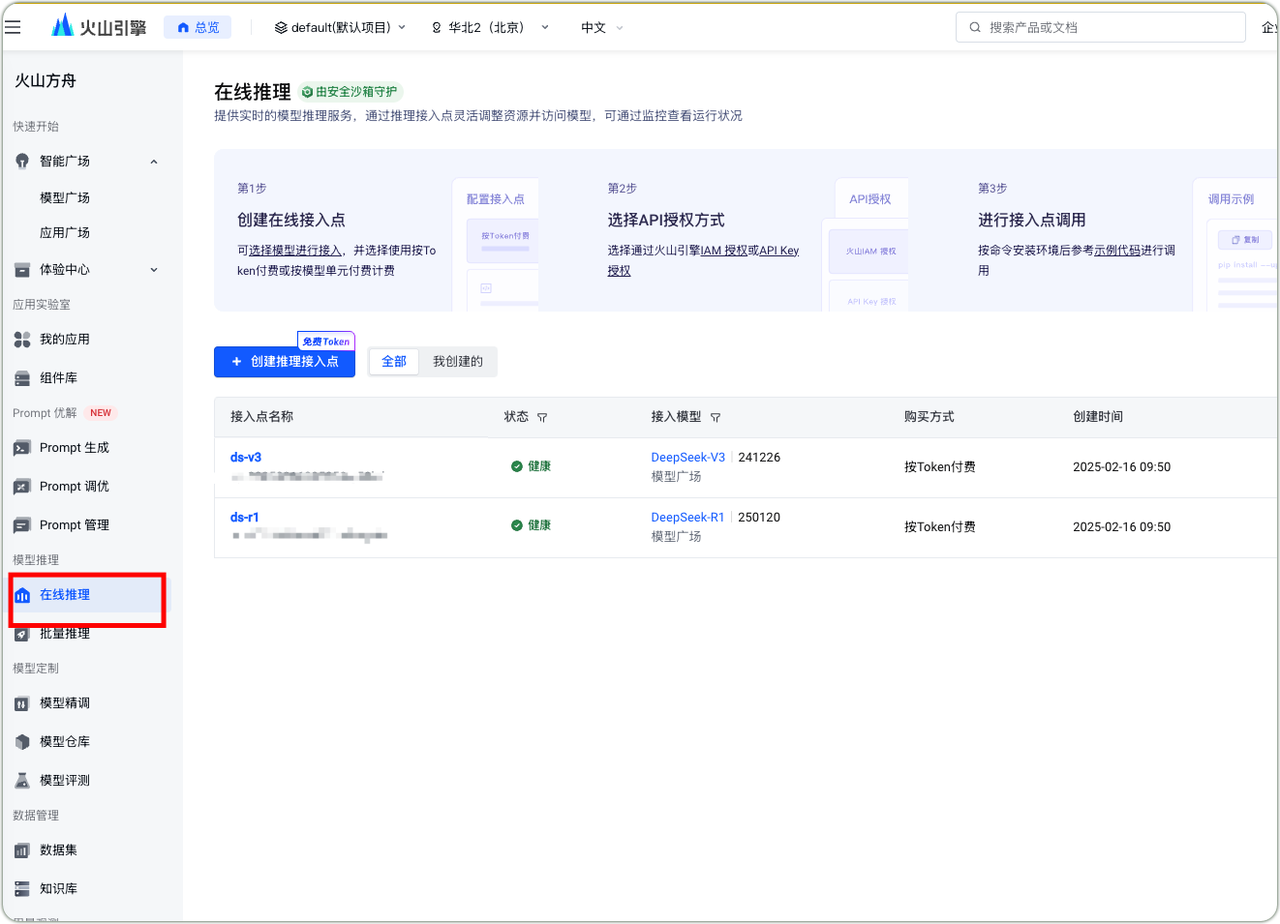
Volcengine Model Access Point
- DeepSeek-R1 Integration with Volcengine
- Enter Volcengine Ark online inference module
- Create inference access point
- Generate API Key
- Determine payment mode
- Complete environment configuration
- Conduct call testing
Gemini API Key
- Visit Gemini management page
- Follow guidelines to create dedicated API Key
Dify Platform Model Integration
- Log in to Dify platform
- Enter model settings interface
- Add DeepSeek-R1 and Gemini models
DeepSeek-R1 Configuration Parameter Example
Taking private integration with Volcengine’s DeepSeek-R1 as an example, configure your custom access model endpoint and API_KEY information in the OpenAI-API-compatible model configuration of the model provider, ensuring all parameter settings are correct.
Volcengine Model Access Point Configuration Volcengine Model Access Point Configuration Parameters
Model Type: LLM
Model Name: Your model access point name
API Key: Fill in your own Volcengine api key
API endpoint URL: https://ark.cn-beijing.volces.com/api/v3
Completion mode: Chat
Model Context Length: 64000
Maximum Token Limit: 16000
Function calling: Not supported
Stream function calling: Not supported
Vision support: Not supported
Stream mode result separator: \n
Quick Creation of Chat Applications on Dify Platform
The Dify platform provides a convenient workflow-style calling method that enables application orchestration without complex coding. During the creation process:
- Set DeepSeek-R1 as the reasoning model
- Set Gemini-2.0Pro as the response model
- Add preprocessing steps such as clearing redundant characters
Dify Application Workflow
For rapid application setup, you can import the pre-debugged compose.yaml configuration file into the Dify platform and modify the DeepSeek-R1 model access point name according to actual needs, enabling quick deployment of the chat application.
Dify DSL File Import
Application Trial and Effect Evaluation
After completing the application creation, conduct functional testing. Taking “how to quickly deploy and use ollama-deep-researcher” as an example, the chat application first calls DeepSeek-R1 for reasoning analysis, followed by Gemini generating the final answer, providing detailed deployment steps from environment preparation (installing Python, pip, Docker, and Docker Compose) to repository cloning, demonstrating the advantages of multi-model collaboration.
DeepSeek-R1 and Gemini Collaboration
Implementing Dify Agent Integration with OpenAI
Private Deployment Model Integration with Dify
For privately deployed models compatible with the OpenAI interface, they can be called by installing the OpenAI-API-compatible component in the Dify tool. During model invocation, precise configuration of model parameters is required, such as model type, Completion mode, model context length, maximum token limit, Function calling, and other options, ensuring compatibility with the target model’s characteristics and usage requirements. After completing the configuration and saving settings, models or agent applications conforming to the OpenAI interface specification can be integrated.
Reverse Integration of Dify Agent Applications with OpenAI
In the Dify platform’s plugin options, search for and install the OpenAI Compatible Dify App, and locate the “Set API Endpoint” function entry.
Dify Model Integration with OpenAI
Select the Dify application to be converted to OpenAI compatible API, input the application-generated API Key, and configure the corresponding application ID in the OpenAI Compatible Dify App to make it work properly.
Converting Dify Application to OpenAI Compatible API Dify Application Compatible with OpenAI Services
After configuration, the Dify application’s API will have OpenAI compatibility.
Taking Cherry Studio as an example, by filling in the converted API address and key during the integration process, you can achieve calling the Dify agent application through the OpenAI interface.
Cherry Studio Application Integration with OpenAI Compatible API
The following image shows an example of the integrated agent application after interface conversion, demonstrating normal chat dialogue functionality.
Cherry Studio Application Model Chat Integration
Related Knowledge Point: The Importance of APIs in Agent Applications
In the process of implementing Dify agent integration with OpenAI, APIs (Application Programming Interfaces) play a crucial role. The workflow of APIs in Web applications is based on a “request-process-response” closed-loop mechanism.
• Client Request Initiation: Web applications (such as browser-based or mobile applications) send requests to APIs via HTTP protocol, including request methods (such as GET, POST), parameters, and authentication information (such as API Key or Token). After receiving the request, the API parses it and determines the required functional module to call.
• API Request Processing and Business Logic Execution: The server-side API executes corresponding business logic according to request type and RESTful design specifications, potentially involving database queries, calling other services, or performing complex computational tasks. During processing, version control ensures interface compatibility, such as user login requests triggering authentication logic and generating session tokens after verification.
• Data Interaction and Response Return: After completing business logic processing, the API encapsulates results in standardized data formats (such as JSON or XML) and returns them to the client via HTTP response.
• Security and Scalability Assurance: To ensure API security, measures such as authentication (OAuth, JWT), rate limiting, encryption (HTTPS) are adopted to prevent unauthorized access and malicious attacks. For scalability, layered design (such as gateway, microservice architecture) and load balancing technology support high concurrency scenarios, enhancing system elasticity and scalability.
API Design
In building ChatGPT-like agent systems, the API architecture acts as a central nervous system, coordinating the collaborative operation of perception, decision-making, and execution modules. • Protocol Specification: Adopts OpenAPI 3.0 standard to define interfaces, ensuring unified access for multimodal inputs (text/voice/image) • Context Awareness: Implements cross-API call context tracking through X-Session-ID headers, maintaining dialogue state • Dynamic Load Balancing: Automatically switches service endpoints based on real-time monitoring metrics (such as OpenAI API latency) • Heterogeneous Computing Adaptation: Automatically converts request formats to adapt to different AI engines (OpenAI/Anthropic/local models) • Stream Response: Supports progressive result return through Transfer-Encoding: chunked The existence of APIs not only enables communication and data exchange between different software components but also greatly improves the scalability and code reusability of desktop and Web applications, making it a core technology for building modern efficient applications.
Conclusion
Through the above operations, we have achieved the integration of multiple models on the Dify platform and their connection with the OpenAI interface. This technical solution provides a feasible path for building efficient, intelligent chat applications, and we hope it can provide valuable reference for relevant technical personnel and developers. Building on this foundation, further exploration of chat application interface interconnection and functionality enhancement with other agents has broad prospects. For example, implementing collaborative work between different agents and dynamically allocating model resources according to task requirements promises to provide users with more personalized and intelligent service experiences. Continuous research and innovation in this field will promote the application and development of natural language processing technology in more scenarios.
Future Prospects:
- Implement collaborative work between different agents
- Dynamically allocate model resources according to task requirements
- Provide more personalized and intelligent service experiences